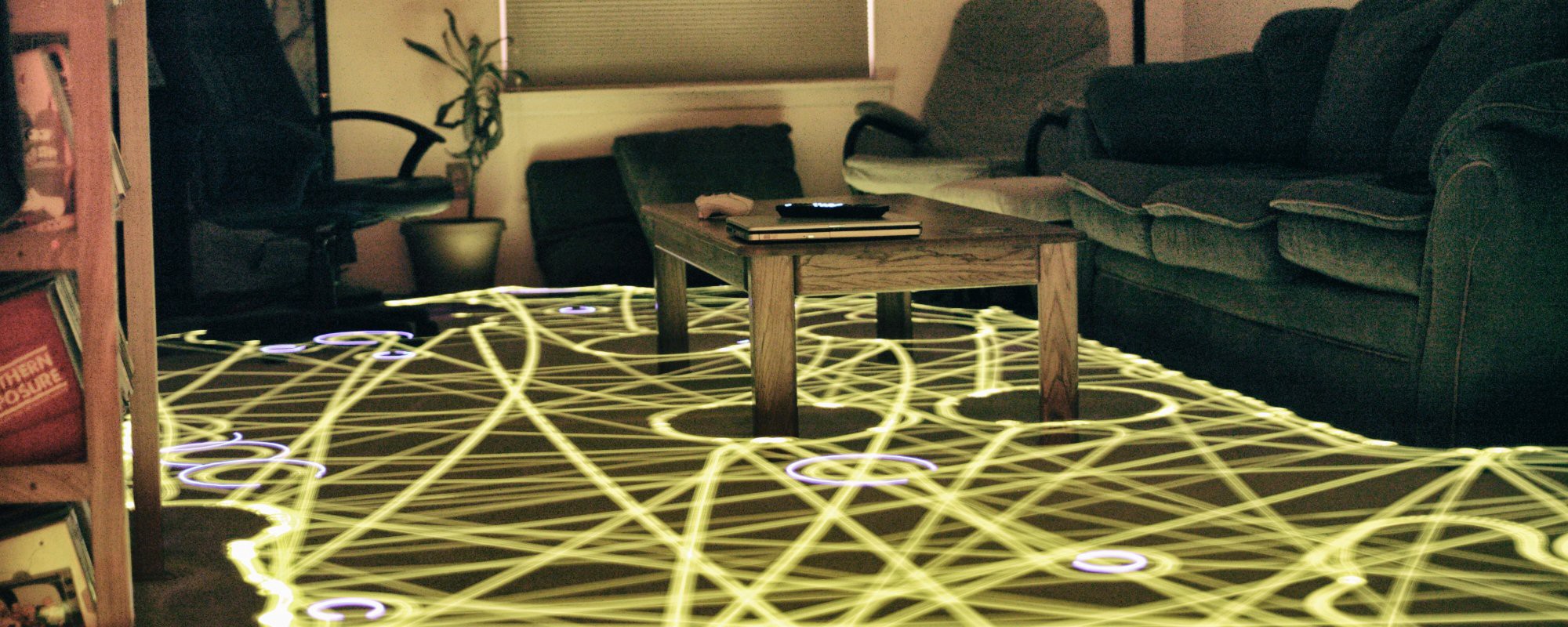
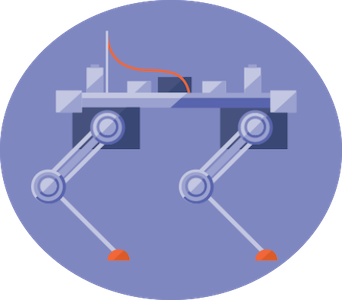
What is intelligence? That’s a big question and scientists are still struggling with it, but they thought they were getting close in the 1980s. By thinking about the brain as a computer, they reasoned they could create something that would think for itself, a true Artificial Intelligence (AI).
The path to this, they thought, included programming machines (called “expert systems“) to make decisions in the same way that people do–by balancing one option against another. That way, they reasoned, eventually we could create one that mimicked the human brain well enough to be intelligent. A complex-enough system could play chess as well as a person and, eventually, think like a human.
Based on the philosopher Thomas Hobbes’s assertion that “reason is nothing but reckoning,” scientists built more and more complex frameworks that could make decisions like humans. Accordingly, in the early and mid-1980s, a wave of companies were founded with the promise of solving the problems of humanity by applying expert systems and related technologies. And they began doing it: NASA was using the technology to help fly the space shuttle, and it was deciding the credit worthiness of American Express customers. Like people, these expert systems could deal with somewhat fuzzy data, weighing factors to make decisions that were more nuanced than a simple yes or no.
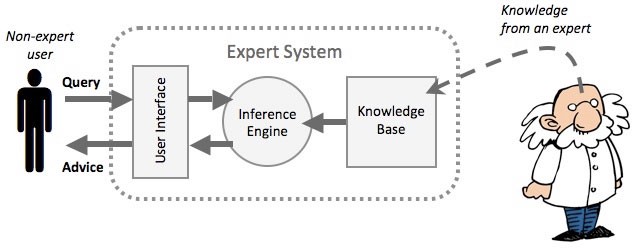
The philosophy behind this technology was called the computational theory of mind. Based on the philosophical idea of symbolic processing, this held that the brain was a computer, in the most general sense of the word. At the basic level you could feed in “symbols” (such as a word or an image) and the brain would process them and create new symbols in response. If a woman in the woods sees a bear, for example, her brain processes this image and tells her to run away. Creating intelligence simply depended on figuring out and modeling the process between input and output, that connection between bear and running away. In short, we just needed to work out the source code of the brain.
Unfortunately, these systems were over-hyped: They were only as good as the rules that programmed them, and if presented with a problem that wasn’t covered by these rules, they could not adapt. To stretch our example, an expert system designed to decide how to respond to a bear would have no idea what to do if it saw a wolf. No matter the type of menacing creature, a human brain figures out that it’s still a good idea to run, but an expert system could not. Companies like Thinking Machines failed, and so did expensive systems designed to revolutionize legal expertise.
Pretty soon, expert systems fell out of favor entirely–and the fall couldn’t have come at a worse time. With the recession of the late 1980s and early 1990s, and associated cuts in government spending, the entire field was seen as tarnished. In fact, some AI researchers coined the phrase “AI Winter” for this period.
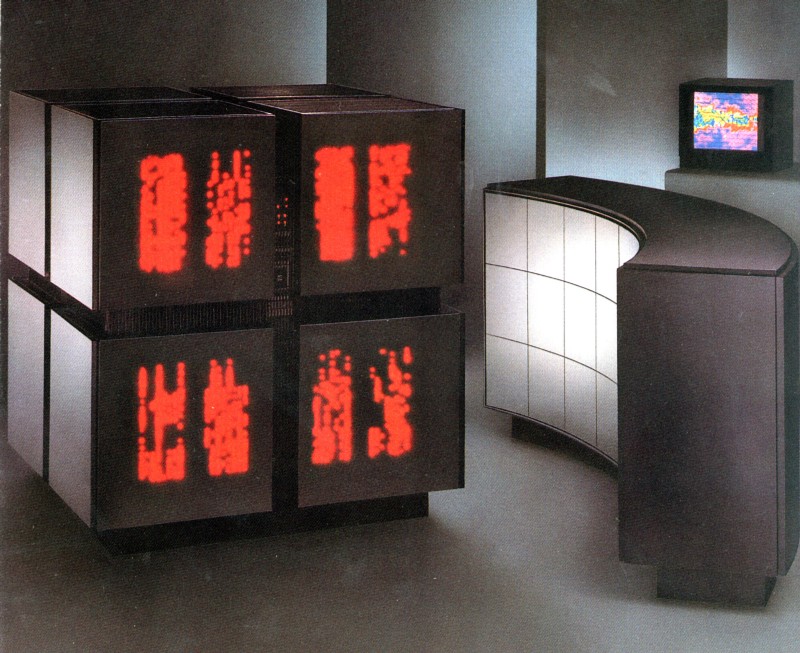

Fortunately, a new approach soon emerged, taking inspiration from animals rather than abstract problems. Its new researchers looked at the physical world, and how insects and other small animals react to their environment. Nobody argued that, individually, an ant was intelligent, but ant colonies could demonstrate very complex and seemingly intelligent behavior, changing and adapting to their surroundings.
MIT lecturer Rodney Brooks was one of the leading figures of this behavior-based robotics movement, and encapsulated this approach in a 1990 paper “Elephants Don’t Play Chess.” In it, he wrote:
The symbol system hypothesis upon which classical AI is based is fundamentally flawed, and as such imposes severe limitations on the fitness of its progeny. We argue that the dogma of the symbol system hypothesis implicitly includes a number of largely unfounded great leaps of faith when called upon to provide a plausible path to the digital equivalent of human level intelligence. It is the chasms to be crossed by these leaps which now impede classical AI research.
Brooks proposed an alternative to the symbols and systems of the previous era, based on simple physical sensors which triggered specific things to happen. A robot would move forward, for instance, until it bumped into something, at which point it would turn and try to move forward again. In the new language he created, this was called a “behavior,” and the overall system was a called a “subsumption architecture.”
His intelligence depended upon a number of different behaviors running concurrently, essentially competing with each other. As the robot moved, these behaviors would interact, and out of this interaction, complex behaviors would arise. To demonstrate this, Brooks built several different robots, the first of which was called Allen. Here’s how it worked:
Our first robot, Allen, had sonar range sensors and odometry onboard. We
described three layers of control implemented in the subsumption architecture.The first layer let the robot avoid both static and dynamic obstacles; Allen would happily sit in the middle of a room until approached, then scurry away, avoiding collisions as it went. The second layer made the robot randomly wander about. Every 10 seconds or so, a desire to head in a random direction would be generated. The third layer made the robot look (with its sonars) for distant places and try to head towards them.
The desired heading was then fed, into a vector addition with the instinctive obstacle avoidance layer. The physical robot did not therefore remain true to the desires of the upper layer. The upper layer had to watch what happened in the world, through odometry, in order to understand what was really happening in the lower control layers, and send down correction signals.

Nobody would argue that the Allen bot was really intelligent, but it demonstrated the premise that Brooks was arguing: Complex behaviors can arise from the interactions of simple ones. Allen, and the many other robots that Dr. Brooks and his students built, behaved like an insect running away from someone–seeking out a quiet spot to hide. And it did so without requiring a lot of computer power to model an insect brain. It ran on very simple (and cheap) processors. Rather than analyzing the world around it with a complex algorithm, Allen reacted to the world directly.
If this sounds oddly familiar to those of you who own a robotic vacuum cleaner, that’s not surprising. Brooks later went on to found iRobot, makers of the Roomba vacuum cleaner, which work similarly to Allen: They react to the environment around them in the most basic way.
This kind of AI research, in effect, led directly to the state of robotics today. Chances are, if you bought a product from Amazon recently, a robot that is a direct descendant of Allen probably picked it out from one of the warehouses. Amazon is only one of hundreds of companies using robots like this.
It was the change in approach in the 1990s that revitalized AI and robotics research after a dark period in which programming rigidity was thwarting progress. As it turns out, programming computers after the human brain and the myriad combinations of abstractions it faces was too ambitious a place to start. Still, it’s a place that we are now better equipped to one day return.
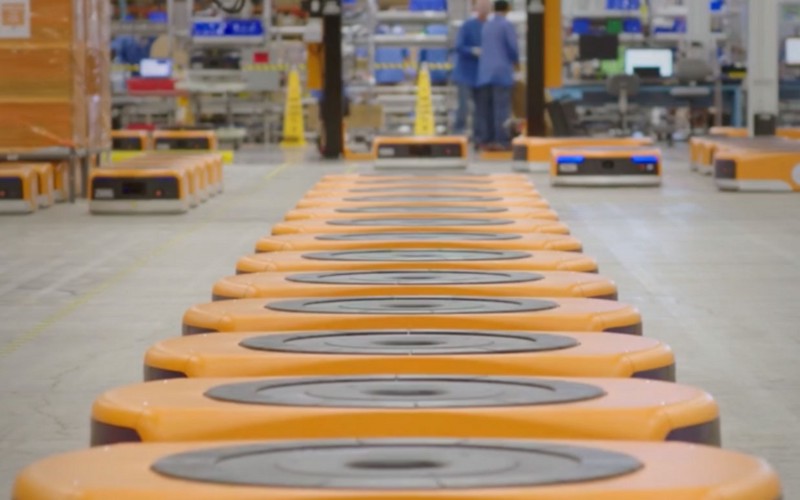

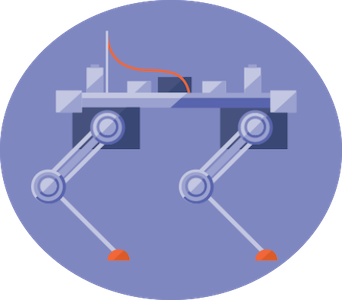
How We Get To Next was a magazine that explored the future of science, technology, and culture from 2014 to 2019. This article is part of our Robots vs Animals section, which examines human attempts to build machines better than nature’s. Click the logo to read more.