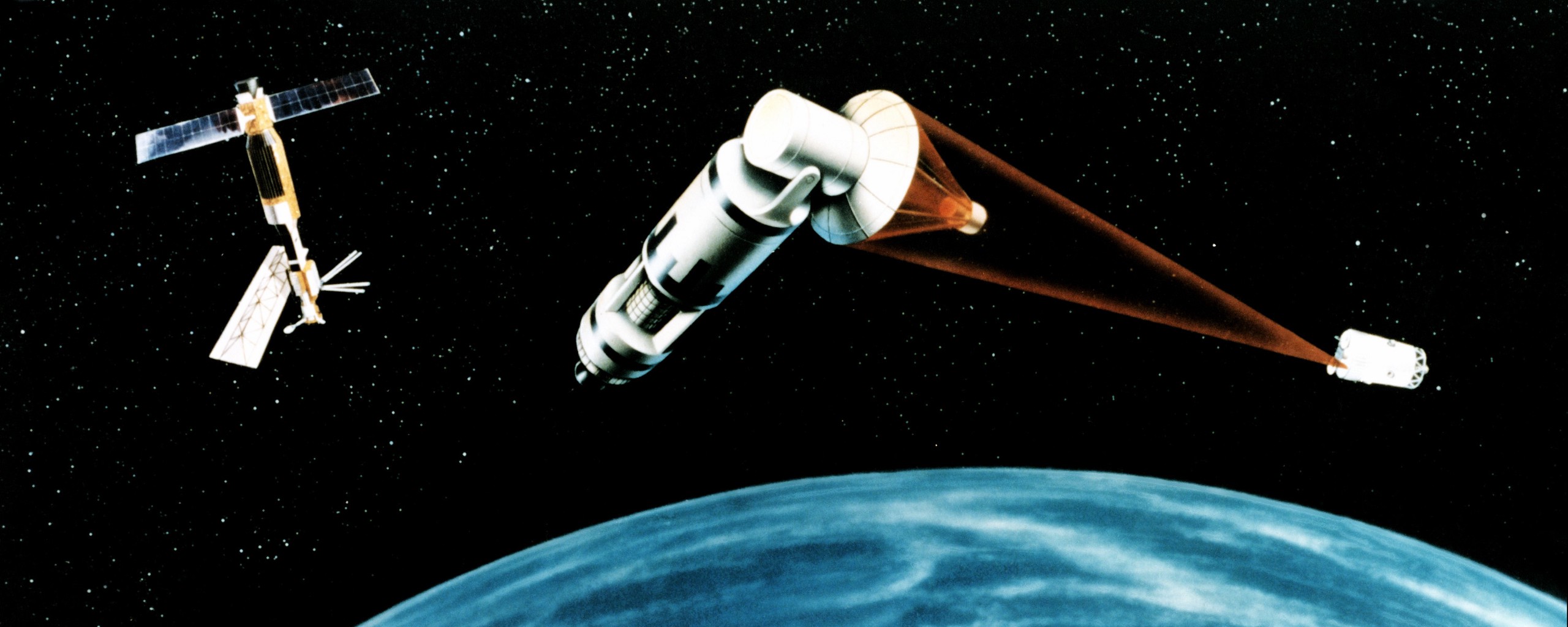
Imagine some scientists from NASA are meeting with the president of the United States. There’s a piece of space coming toward us; it is rocky, and icy, and big, and the risk of it hitting the Earth is much larger than anyone is comfortable with.
Even if there’s time to act, there won’t be much of it. What can we do? Where did it come from? How come we didn’t spot it until now? What’s the best course of action to take?
There are scientists around the world who have dedicated their careers to answering these questions–painstakingly scanning the skies, tracking meteorite landings, and cross-referencing different rock types with the known asteroid classes of the solar system to try and figure out what’s out there.
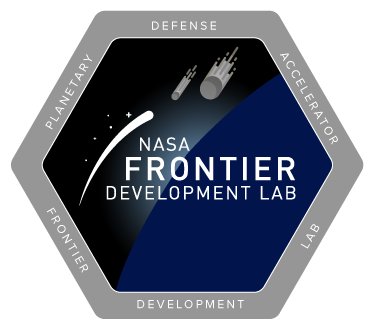
NASA’s Frontier Development Lab (FDL) is pioneering a new approach, however, which could dramatically improve our chances of responding to a threatening object hurtling toward us.
We’ve turned to artificial intelligence and machine learning–in essence, teaching a computer how to learn by itself, so that it becomes better and better at a set task without needing a human programmer to fine-tune it. It’s not the all-encompassing AI Skynet from the Terminator movie series, but it’s along the same lines. Just ignore all that business with the nuclear bombs and time travel.
Similar methods are being used in almost every field, often with striking success–from diagnosing cancer patients more quickly in hospitals, to predicting future human behavior from video clips in AI labs; and from companies like Apple, which are making personal-assistant AIs more intuitive and helpful than ever before, to improving real-time translation with services like Google Translate. Machine learning is quietly reshaping much of our world, so it makes sense to use it to deal with problems that occur outside of it as well.
“This new approach of combining big data, powerful computing, and machine learning has the potential to expand more complex aspects of asteroid deflection,” said James Parr, FDL’s co-director.
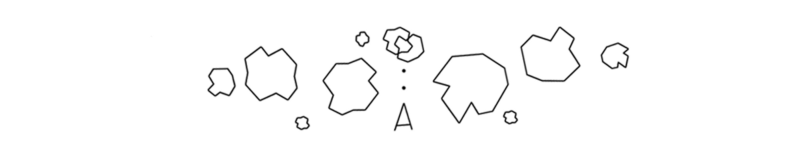
Even if we don’t know when it’ll happen, a large asteroid will eventually collide with Earth–and not just because of the scars from previous impacts that are scattered across the globe, providing evidence of a statistically inevitable repeat of history. We know that because we’ve been tracking a lot of them for more than a decade. In the 1990s, NASA began focusing on so-called Near-Earth Objects (NEOs) in response to a growing realization that anything could hit us, at any time, without much warning. Any object that comes within 45 million kilometers of us–just under a third the distance from the Sun to Earth–gets classed as an NEO, and rated for risk accordingly.
The global database of known NEOs–all 14,761 of them–is kept by the IAU’s Minor Planet Center. The most interesting ones, though, are a subset: the Potentially Hazardous Asteroids, or PHAs. They’re the ones that come within 0.05 AU of Earth when crossing its orbit, or just under 20 times the distance between the Earth and the Moon, and which are more than 140m across. We’ve recorded 1,726 of those so far, and we know there are more. Astronomers currently believe there to be roughly 4,700 in total.
The risk of a planet-wide extinction-level event happening because of an asteroid or comet strike is negligible–that would require objects larger than six miles [10 kilometers] in diameter, and we’re pretty confident we know both where they all are and that they won’t cross paths with the Earth any time soon. The real risk is a PHA that’s small enough to avoid detection, but still powerful enough to kill.
Think back to February 2013, and the Russian city of Chelyabinsk–more than a thousand people were injured from the shockwave of an asteroid exploding 18.6 miles [30 kilometers] above them. That meteor came in at a shallow angle, from the direction of the Sun, making it impossible for astronomers to have spotted it in advance; and it was only 26,455 pounds [12 tonnes] and 65.6 feet [20 meters] wide. If it had come in at a more direct angle, it could have killed people. Something only slightly larger–say, over 500 feet [or a couple hundred meters] in diameter–could easily wipe out a whole city, or much of an entire country.
NASA currently estimates that fewer than 10 percent of NEOs smaller than 1,000 feet [300 meters], and less than one percent of those smaller than 330 feet [100 meters], are known. Those smaller objects, those “city-killers,” are the focus of NASA’s Grand Asteroid Challenge, which prompted the creation of the FDL.
“[It] was established to do two things,” said Parr. “Firstly, apply machine learning techniques and technologies to the challenge of Planetary Defense–specifically, PHAs or Potentially Hazardous Asteroids. Secondly, to demonstrate a platform where significant breakthroughs could be “‘industrialized’ over an accelerated timeframe, in a way that is useful for America’s space program.”
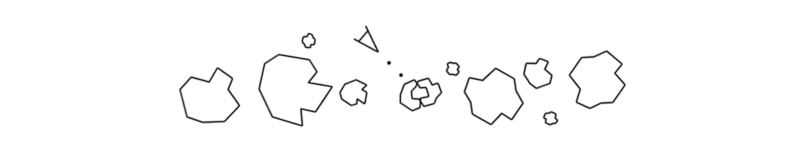
Let’s go back to our hypothetical President Clintrump to see how it works–how, exactly, machine learning has practical benefits for protecting the world from asteroid strikes. There are three specific things we must know to have any chance of launching a mission to intercept and redirect (or even destroy) an asteroid: what it’s made of (which gives you its mass), what shape it is (which gives you the center of mass), and what the best way to deflect it is in the time available.
The time it takes to figure out these things could be the difference between life and death for hundreds, even thousands, of people. Machine learning can help reduce that time; it also massively improves our ability to analyze different asteroid threat scenarios. More analysis means a better understanding of which deflection plans are most appropriate for which kinds of asteroids.
Take the first point: Asteroids group together in “families” throughout the solar system, “like disaggregated planets,” explained Parr. If you spot a meteor coming down, and collect what’s left (provided it doesn’t burn up completely), then you’ll have a sample of that meteor’s family group. There are roughly 25 different families that astronomers distinguish by the spectra of the light they reflect, though they can broadly be split into three main groups: rocky, organic compound-rich C-types; stony, silicate-rich S-types; and iron-nickel metallic types. There are innumerable meteorite samples in collections around the world–the major challenge is in matching those recovered samples with their distant asteroid relatives throughout the Solar System.
(As an aside, to avoid any confusion from hereon out, an asteroid is what we call the rock when it’s in space; a meteor is when it’s falling through the atmosphere; and a meteorite is what doesn’t get burned up on entry and lands on the ground.)
“For 200 years the fields of meteorites and asteroids have developed separately,” Parr said. “Many meteorites are in collections, in 40 categories, but it is not known how they related to the 24 types of asteroids, or the dozens of asteroid families. Fireball tracking networks have been established around the world, tracking about 800 meteors [so far] to determine a source orbit and an impact target region. But only 27 meteorites have been found that can be linked to a source orbit [from those]. Meteorite searches in target regions can require hundreds of hours to possibly find one meteorite.”
This is partly what makes samples recovered directly from asteroids by probes–like the upcoming OSIRIS-Rex mission, due to launch in September–so invaluable for providing that direct link between a meteor class and its origin orbit.
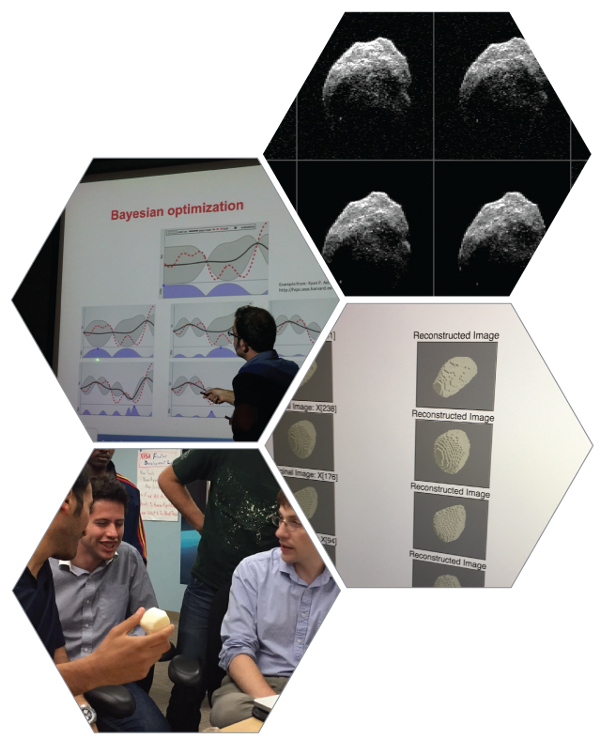
To solve this problem, the FDL team–supported by nVidia and Autodesk–adapted an Uvionix rapid response drone, so that it will fly into action when a meteor is spotted, and find and retrieve the small meteorite on the ground.
They used six machine learning models to design its program, training it on 25,000 images of meteorites and a larger 15 million image library of other objects, so that it can spot meteorites on the ground with a 0.7 percent false positive rate. (Parr said that’s still too high, though, and that it’s still too computationally intensive for field deployment.)
By contrast, figuring out an asteroid’s shape is another kind of time-intensive task, he said: “[It’s] a labor- and computing-intensive process, requiring long computer runs using a 50K-line legacy code, and about four weeks of human-guided iterations to get one asteroid shape. Asteroid shapes are critical for asteroid deflection techniques–as any mitigation plan needs to know the center of mass.” Even if an asteroid is too close to stop in time, knowing its shape and mass will help in predicting the nature of its impact. Machine learning applied by the FDL has reduced those weeks of computation to “milliseconds,” Parr claimed.
Machine learning has also proved incredibly useful in solving the third problem–that of working out how to deflect a PHA. Up until now, only limited orbital models have been used to estimate what the best method is to stop it. That could be blasting it with a nuclear bomb, smacking it off course by crashing a probe into it, or simply attaching an engine to it and driving it out of harm’s way. To improve on that, the FDL built an analysis model using machine learning to crank out over 800,000 simulated orbits, a huge improvement in modeling complexity.
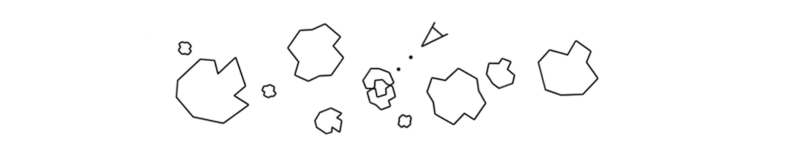
There’s something exciting about using artificial intelligence to help defend our planet from a cold and indifferent asteroid. It’s going to be fascinating to see how else the space program benefits from this approach to solving problems, from spaceship design to working out better ways to grow crops.
“As science fiction writers have known from the beginning, machine intelligence is a critical enabler of space exploration,” said Parr, “whether it is remote data gathering and analysis, robotics, decision support, or autonomy. Ultimately, we need HAL. We perhaps don’t need some of his personality traits, but we need his capabilities.
“We’re entering an era where these science fiction ideas are now reality. The GPU revolution is already powering the next phase of space exploration in the same way the CPU microprocessor enabled NASA’s missions to the Moon.”

How We Get To Next was a magazine that explored the future of science, technology, and culture from 2014 to 2019. This article is part of our Above & Beyond section, which looks at our understanding of the universe beyond Earth. Click the logo to read more.