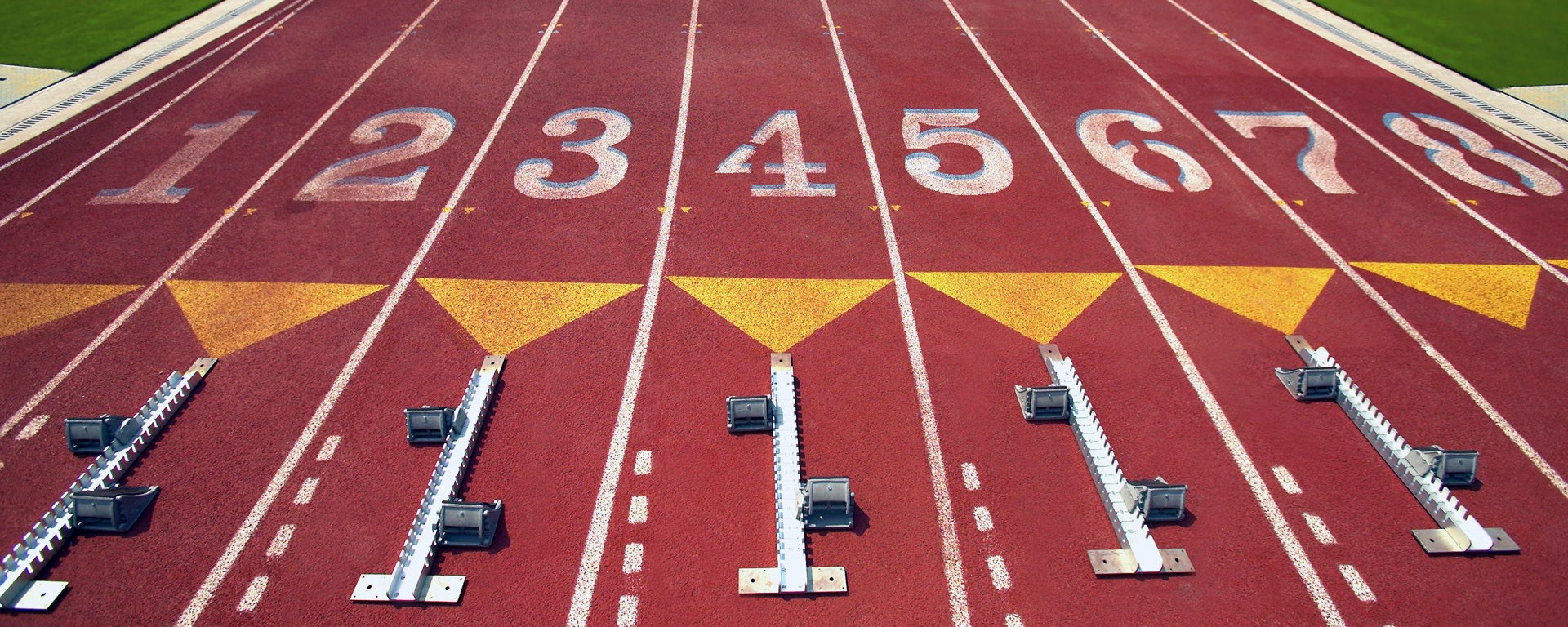
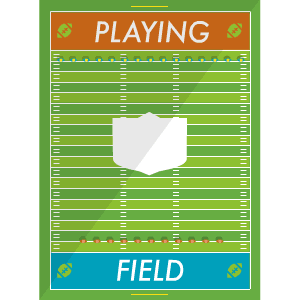
Audrey seems like a regular kid by 2040’s standards. We did all of the regular kinds of tests–we only wanted what was best for her, anyhow, as any parent would.
How my and my partner’s bodies grew and aged, how they transported oxygen, how they respond to stress and stimuli–all of this can be predicted in our child. Before she was even born, we had a corpus of data; as she grew in utero, we knew what kind of oxygen transportation system she had and how it could interact with her muscles, bones, ligaments, and tendons.
The doctors tell me it’s a variant of some version of machine learning, called “semi-supervised learning.” They take data and construct an accurate learning model–and we can catch all of that data, and really start to change and pivot as she grows. We can tailor something to her specific growth schedule. The daily data collections, from weighing to blood collection, really don’t take up that much time. It’s worth it, too. Wouldn’t you do anything for your child?
By starting her early, she can probably be an Olympian. By better understanding her body, we can help her train and recover with an algorithmically designed workout regime specifically tailored to her.
Why waste time on swimming if she’s genetically predisposed for something else?

How well can we make the human body work, before there’s even a problem or trauma? And how well can we train and recalibrate the body and brain toward optimum performance? The above is more of a dystopian and myopic view of how to apply machine learning algorithms, which are emerging inside medicine today, to our daily lives.
So much of a specific health plan feels like puzzle pieces fitting together. What’s the right cocktail of antibiotics to prescribe? Does it take into account allergies and preexisting conditions the body has, as well as how well similar bodies have responded to that prescription?
Oncology has started to use machine learning applications that are helping to discover and identify patterns within complex datasets. They’re able to effectively predict future outcomes of some cancer types, and they can also be used to create much more highly personalized care. Genetic makeup is a large-scale dataset, and this kind of predictive medicine–which was once the future–is quickly becoming a reality in targeting aggressive forms of diseases.
Recently, advances in algorithmic genomic testing have started to look at and predict which kinds of genes are better for certain kinds of sports. If Audrey tests as a low aerobic responder, running would be out of the question. Perhaps body building would be better? What would it mean to know that, though, as an athlete or a child?

When I was young, the world was already used to artificial intelligence winning at board games, chess games, and TV show competitions. That soon gave way to therapeutic chat bots and robots assisting the elderly. By my early teens, in the 2010s, a consultancy predicted the rise of artificial intelligence in medicine. People assumed that meant robots replacing doctors, but that was far from the truth–and in 2040, it still is.
Instead, medicine began experimenting with how to use machine learning to predict drug recovery times and pinpoint diagnoses that involved doctors talking to patients to surface information first. I know this sounds ridiculous, but really, that’s what people did back then! Doctors looked at photographs of different ailments and made diagnoses based off shading, perceived texture, location, and preexisting images of that ailment. Unlike science fiction led us to believe, there were no robotic nurses–just nurses using different kinds of tools.
Ten years later, though–sometime around 2020–medicine started to get less personalized and more customizable. (“Optimizable” is probably the better word, actually.) Because questions of how best to cure a specific sickness seemed easier to answer, patients and doctors started to ask more about general well-being, and using machine learning to help augment the human experience organically–to tailor-make a workout regimen, say.
Around three or four years of age is when the more in-depth genetic testing occurs now, along with personality tests. We want to make sure Audrey will enjoy the sports she will participate in. It’s not uncommon to do a stress test–really, we’re just seeing how well she responds, based off the projections. What we learn from Audrey can help other children in the future. The doctors say it’s part of a training corpus, and with all of our input the algorithm will get better as it’s guided by real feedback.
It’s not just about choosing what sport is right based off of one’s body type anymore; it’s about choosing how to use that body to train effectively for years and years. If you’re predisposed to arthritis, for example, what are the best combinations of training regimes, stretching, and diet to make sure you stay as fit as possible, for as long as possible?
The system can know that, and the system can guide us with the input of a trained physician and dietician.
When I was a child, this was all in beta form. It was a specific kind of privilege–I was going through normal athletic training, but in a whole new way. What could the combination of my genetic makeup, plus my physical body, bone density, flexibility of muscles, and my actual shape determine for sports?
I came from a long line of athletes and my parents wanted me to shine. What kind of exercises should I engage in to target my muscles and stamina? How did my particular diet play into effect, and what about how my gut reacted to food? All of the sudden, I had a “me”-specific food and workout plan.
We wanted that same kind of training for Audrey. We were lucky that after a generation, it became much more widely adoptable. It became the status quo.

It’s something to think about. The systems that doctors and technologists are designing right now use real data, but algorithmically parsed. It’s somewhat experimental, but like all machine learning it depends on repeated use and offers real, actionable results.
Machine learning algorithms are working to combine large bodies of cancer data with the genetic makeup of patients, to try new kinds of cures that would work with the specific kind of cancer the patient has.
Machine learning in medicine allows for highly personalized treatment. These are not one-size-fits all solutions, but something tailored to both a person and their cancer. When these prove to be successful enough, doctors and patients will inevitably start to wonder about using machine learning and medicine to improve daily life outside of the cancer ward.
If we’re going to use technology to help cure incredibly vicious and high-stake diseases, then can we not take those same systems and apply them to more holistic medical approaches? If our genetic makeup can show how resilient our muscles are, how much oxygen our body can process, and if our body is designed for running, then why not use medicinal algorithms to help craft a better sports performance? But maybe a more important question is, should we? Should we map our genomes and algorithmically test our own DNA for outputs like sports? Where does that data go, and can we determine if it’s being ethically parsed, saved, and fed into the algorithm itself? There’s so much good that can be done with machine learning and genetics, especially for aggressive diseases like cancer. But it’s important to think about the flip side: If we are going to use these same sort of programming structures for holistic medicine, or even more daily concerns, are we creating systems that accidentally surveil or discriminate? When our data is about our genes, and the goal isn’t something as clear cut as eradicating cancer, what is our data doing?

I sometimes forget that we’ve been using this kind of testing for 30 years, and now it’s normalized. So I try to remember, with every aspect of what we can test–are we giving Audrey a choice?
A choice in what she wants, even if it could genetically, algorithmically, be proven to result in failure? Audrey may not like sports, but we want to give her every shot at success we can, algorithmically speaking.
I had these questions myself when I met my partner and we started talking about kids. After all, it wasn’t just about eradicating diseases. What could we do to make ourselves better? What could we start immediately with our kids? Based off my genetic makeup, what tasks should I complete by which age, and what and when should I eat, to achieve the best outcome my genetics dictate?
How can I use artificial intelligence to become a better version of myself? Isn’t that a question we should all ask ourselves when we strive for betterment?
Like every other family, we had Audrey checked out–genetically speaking–before she was born. I mean, you just should, especially if you can! Why not know what you can do to make your child the best possible version of itself? My partner and I did it ourselves, too.
Have you done it yet? You really, really should.

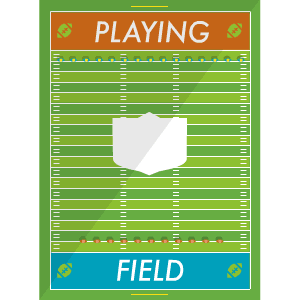
How We Get To Next was a magazine that explored the future of science, technology, and culture from 2014 to 2019. This article is part of our Playing the Field section, which examines how innovations in sports affect the wider world. Click the logo to read more.