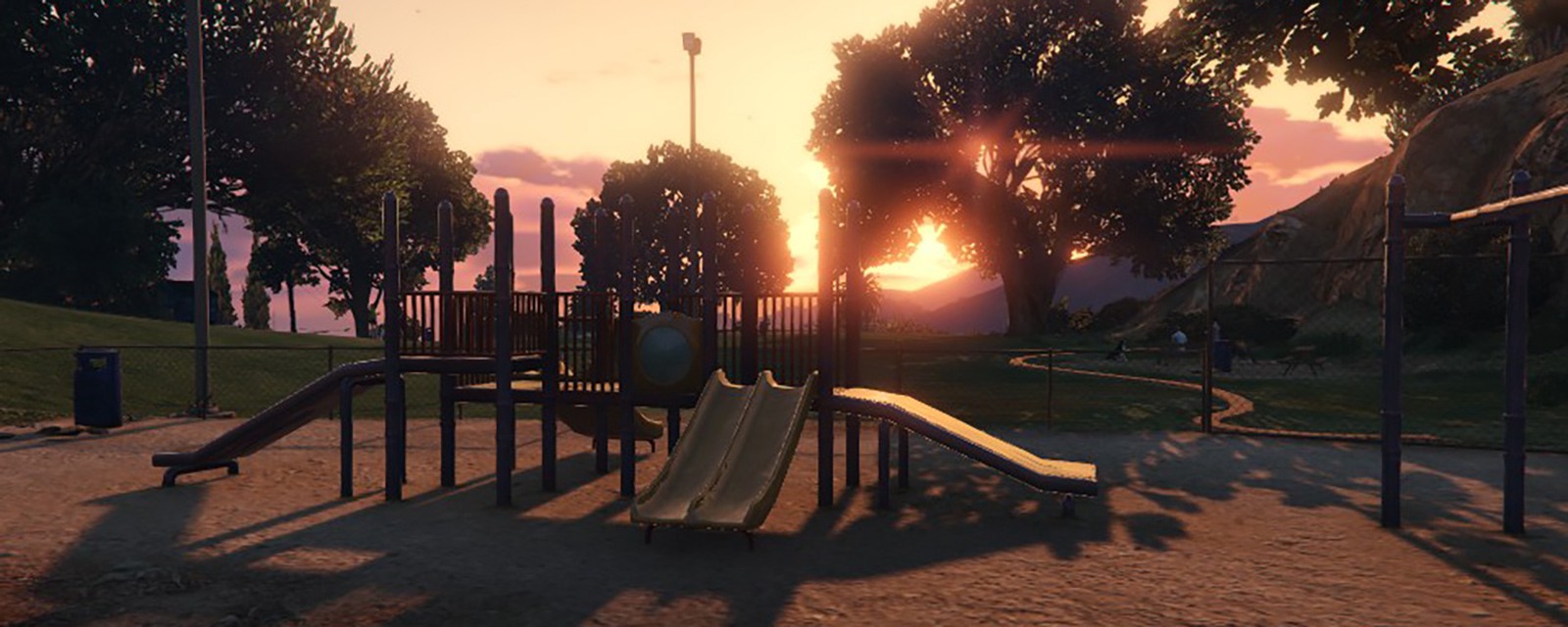
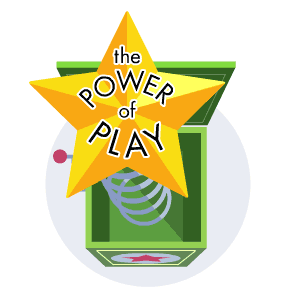
Childhood development experts are reaching a growing consensus on the value of play. As kids transition into adulthood, the ability for them to experiment in a safe environment has major benefits to emotional and cognitive development.
Now AI researchers are beginning to recognize the same thing–that their creations benefit just as much as children do from a playground, where they can learn how to behave in the world. For kids, that usually involves a jungle gym, a sandpit, and maybe even a slide. For AIs, that playground is found inside videogames.
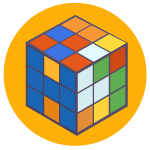
It’s been a while since Arnold last shot anyone. After searching the basement for potential victims, he bounds up the stairs to the vast and forbidding control room. It’s there that Arnold spots a fellow space marine, Ivomi, standing by a computer panel. Arnold sprints past his quarry, almost close enough to reach out and touch him, then crouches by another terminal, before doubling back. He fires his pistol. Despite the large quantity of blood gushing from his side, Ivomi seems unaffected. Arnold decides to run away.
This scene of random violence–one of many–was recorded at ViZDoom, a competition that Marek Wydmuch, one of its co-creators, likes to refer to as “the biggest LAN party for AIs in history!” The premise is simple enough: Teams representing institutions from universities, Facebook, Intel, and more program artificial intelligence systems to fight to the death against one another in a modified version of the classic 1993 shoot-em-up Doom.
The catch was that each AI could only navigate by interpreting the game’s visual data, which offered each team a set of difficult training challenges. The systems would need to be able to differentiate walls from open space, and enemies, too. They’d also have to judge distance, as the in-game rockets have a blast radius that can harm the user as well as the opponent, and devise tactics to maximize the chances of eliminating their rivals while avoiding return fire.
“As far as I am aware, there were no studies that used raw visual information from a 3D first-person shooter game before we started the ViZDoom project,” says Wydmuch. “It opened a new field for research, in that the competition proved that deep-reinforcement learning works in 3D environments, which significantly increases its real-life practicality.”
In fact, ViZDoom is just one example of games shaping the future of artificial intelligence. As the realism of simulated worlds within videogames has grown, so has the awareness among AI researchers that developers have–unwittingly–created ready-made training environments for artificial intelligence. In short, the software that powers the likes of Arnold and Izomi–just two bot-controlled, bloodthirsty space marines–could prove to be the direct antecedents of our future robots, intelligent assistants, and driverless cars.
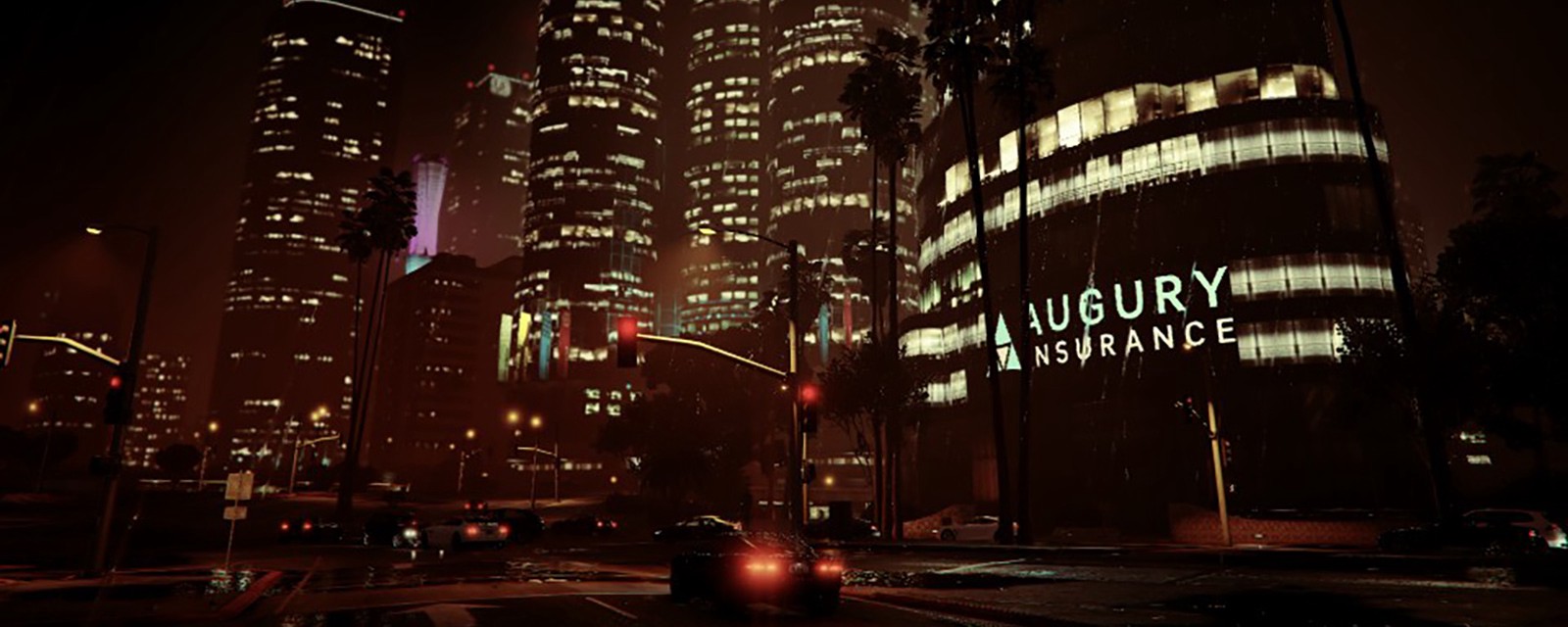
In truth, games have always proven to be far more than the sum of their parts. Fundamentally, they give people the chance to express and pursue experiences they would not otherwise encounter in their daily lives–from blowing up space marines in Doom, to buying up property on a Monopoly board, or scrambling for territory on the football field. In that sense, the mastery of one game or another by individual players has always been regarded as an easy shorthand for a person at their physical or intellectual zenith.
From that perspective it’s obvious that AI scientists would see games as the most effective platform in which to prove the utility of their creations. The barely veiled subtext of this pursuit has always remained the same: If a machine can beat a human opponent in a game not only once, but ad infinitum, they must be greater at the specific task than the greatest humans. What else might they achieve?
Until 2013, the answer was “not a great deal.” But when the massive processing power of IBM’s Deep Blue supercomputer was brought to bear on the chess board against Garry Kasparov, the grandmaster’s dazed and confused expression in defeat hailed a major breakthrough for machine learning. Yet, in the end it merely showed the depth to which a machine could master one single task. If Kasparov had challenged Deep Blue to a game of checkers, the results would not have been as astounding.
So AI researchers began to pursue a new goal–an algorithm capable of playing any game without prior knowledge of how to win it. The first clear demonstration of this capability would come in December 2013, when Demis Hassabis and his team at the AI research startup DeepMind Technologies presented software capable of mastering Pong, Enduro, and Breakout. All the algorithm had access to were the controls, the display, and the score, with instructions to maximize the number of points any way it could.
At first, the AI’s performance was poor; in Breakout, the algorithm couldn’t efficiently move the cursor. After a few hours, though, the program had not only learned how to master the game, but had worked out how to dig a tunnel on the side of the wall of blocks, trapping the ball behind them and letting the game do most of the work. “The designers of the system didn’t know that strategy,” said Hassabis, speaking to Wired.
What DeepMind had demonstrated was the potential of a machine learning technique known as “reinforcement learning,” by which the algorithm at the heart of the system made new choices based on feedback from previous decisions. This, in turn, was underpinned by a crude replication of the network of neurons in the human brain known as a “Deep-Q Network.” In March 2016, Hassabis and his team followed up with an algorithm capable of outplaying the world champion of Go, a board game with vastly more possible moves than there are atoms in the universe.
Yet while the debt that artificial intelligence owes to games is clear enough, DeepMind and its like have not moved beyond the level of demonstration. For Hassabis and his colleagues, games are still, for the most part, the training wheels. “Games are just our development platform,” he told PCGamesN in an interview. “It’s the fastest way to develop these AI algorithms and test them, but ultimately we want to use them so they apply to real-world problems.”
Hassabis and team are working on that, but progress has been slow. While DeepMind announced a partnership with Moorfields Hospital earlier this year to automate the process behind diagnosing certain eye conditions, the AI’s role in that case is merely the interpretation of big data. Set it out in the world, though, and it would still be blind.
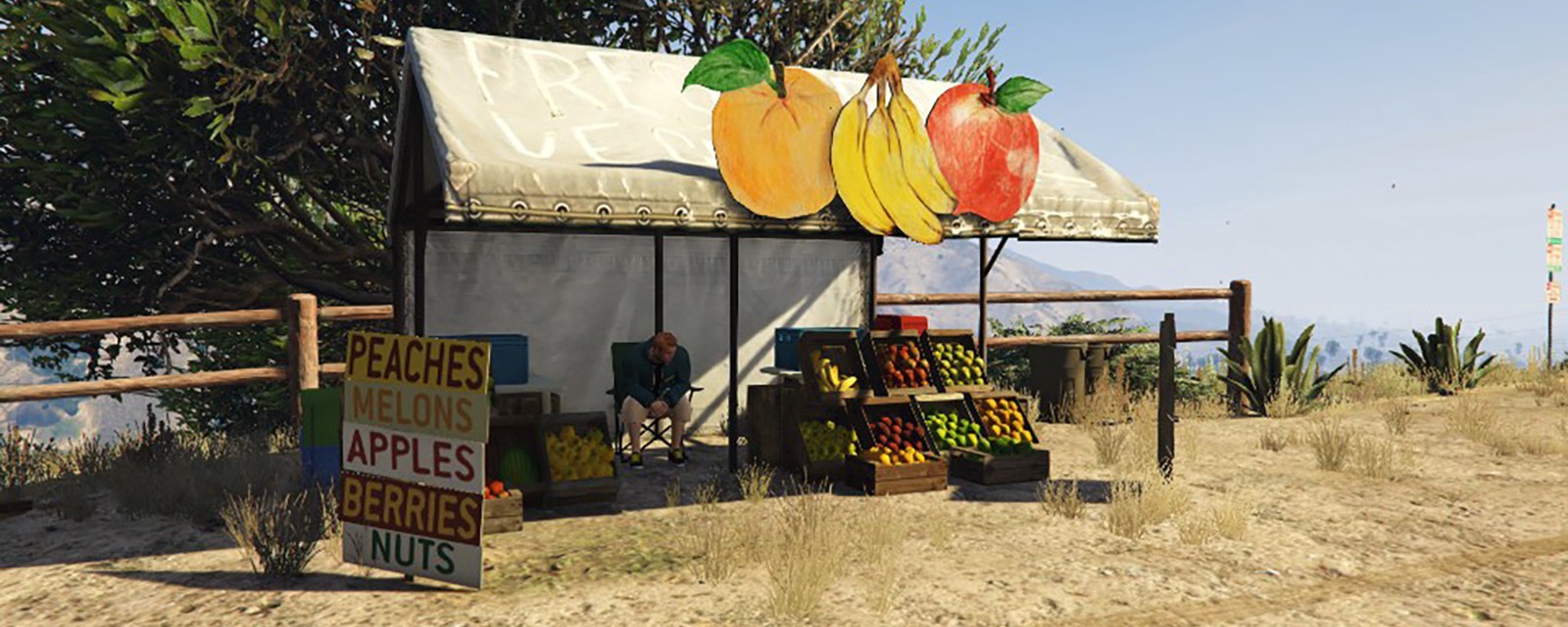
That, it seems, is why a team of AI researchers from TU Darmstadt and Intel Labs have spent this summer playing Grand Theft Auto V. In late 2015, Stephen Richter and his colleagues were searching for a way to automate the tedious process of collecting and labeling the many thousands of images used to train the visual machine learning algorithms for autonomous vehicles. Indeed, the task of collecting such a vast amount of image data puts the option of researching visual machine learning out of reach for many academic institutions. One of the reasons Tesla has coped so well with its Autopilot driving systems is because of the vast amount of sensory data being collected by its fleet of cars, day in and day out.
When Richter and his colleagues devised a visual learning algorithm capable of automatically annotating imagery, they needed to be able to test it within a convincing facsimile of the real world. Knowing that other such teams often resort to creating 3D simulations from scratch, they recognized the potential of open-world videogames. One in particular, with its rough-and-ready evocation of Los Angeles and its surrounding counties, stood out.
“GTA is the most sumptuous, the most realistic, and the closest game to [our] existing datasets,” Richter tells me. “We have shown people photos from the real world from the cityscapes dataset and also from [the game], and it took them a moment to figure out which one is which.”
Using a modified version of an open-source program called Renderdoc, the team downloaded one frame per second during a set period of gameplay, from the point of view of a car dashboard. The resulting image data was then intercepted by the visual learning algorithm, which sat between the computer and the game itself.
The results were astonishing: The algorithm labeled 25,000 of the images extracted from GTA V in under 50 hours. “Three orders of magnitude faster,” the researchers said, than if attempted with real-world images. None of this, they say, could have been possible with manual labeling.
What’s more, Richter and his colleagues believe that this line of research could work for more than just autonomous cars. “It’s relevant for all kinds of vision tasks where you’re looking for realistic data,” he explains. “There’s interest in creating synthetic data for human pose estimation, other types of 3D scene understanding, estimating depth from an image, and probably object detection [too].”
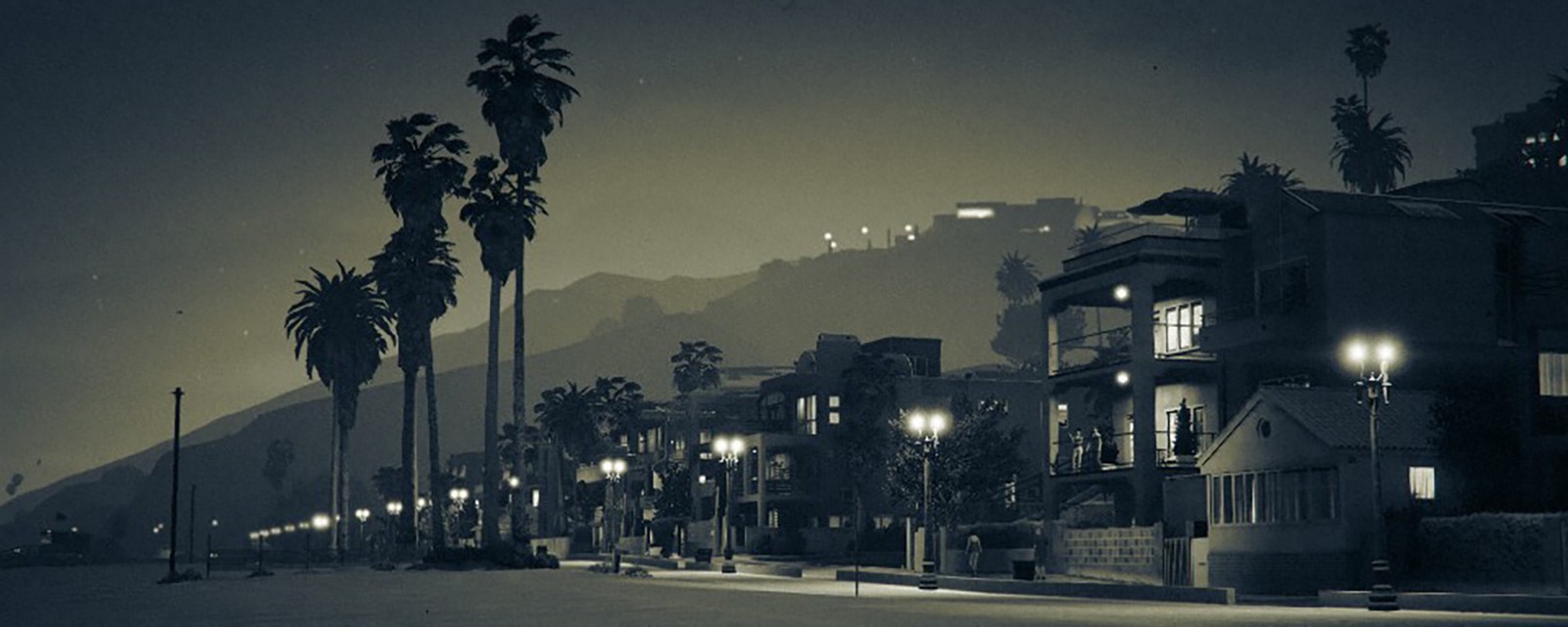
The work conducted by Hassabis, Wydmuch, and Richter proves that games continue to be the best place to test many specific AI applications. But there’s at least one team of researchers working even harder on the crossover between gaming and AI research. Katja Hofmann and some colleagues at the Microsoft Research Lab in Cambridge, United Kingdom, are working on erasing the line between the two in an effort code-named Project Malmo.
Malmo is the first platform that uses Minecraft as a testbed for what Hofmann terms “collaborative AI.” Comprised of a system that, according to Microsoft, “helps artificial intelligence agents sense and act within the Minecraft environment,” Project Malmo is a sandbox for developers in which a broad range of AI experiments can take place. While Minecraft‘s iconic blocks don’t lend themselves to complex research into visual learning applications, they’re the perfect environment for experiments into navigation or assessing the limits of collaboration between individual AIs in tasks of varying difficulty.
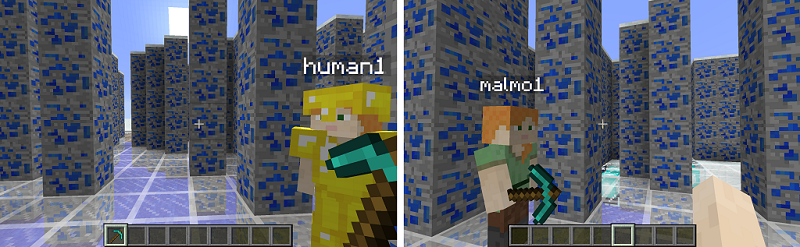
“We needed an environment where we could experiment much more quickly, but where we maintained [a high level of] interactivity,” explains Hofmann. “This focus on having to have an interactive setup really lends itself to be explored in a game context, because you put an agent in an environment. It has to interact with that game world, and it learns, over time, to achieve certain goals within that environment.
“When we started this, of course we knew about [DeepMind’s] work in Atari games, but what we were looking for was something where this interactivity is much more open-ended. Personally, I want to push towards AI technology that can learn to collaborate with people, that helps them to achieve their goals.”
Hofmann envisions applications in the development of intelligent assistants and robotics, but remains hesitant when it comes to specifics. The platform, she explains, has only been running for a few months, and it was only recently that the source code was made public. Even so, Hofmann says that Project Malmo has already acquired a vibrant international following of university students, professors, tech company employees, and Minecraft enthusiasts from as far afield as China, New Zealand, Malaysia, Brazil, and India.
It’s impossible not to get the sense from Hofmann that this area of research is not only innovative but beginning to flourish. “A lot of the machine learning, [the] kind of interactive learning approaches that we see today are extremely data-hungry, and improving them further will require large amounts of data and very high bandwidth and experimentation. And for that, games are just fantastic,” she says.
“I think what’s underappreciated is the power of these platforms to really increase the speed of innovation, to allow us to very rapidly make progress in the underlying learning technology, and the fact that this will translate into better algorithms for real-world applications.”

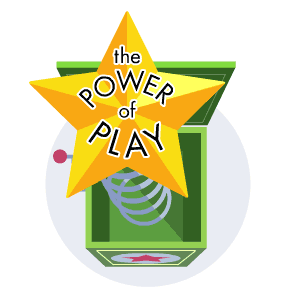
How We Get To Next was a magazine that explored the future of science, technology, and culture from 2014 to 2019. This article is part of our The Power of Play section, which looks at how fun and leisure can change the world. Click the logo to read more.